The challenges of using true demand through the supply chain
Sell-out data can transform demand forecasting, optimise supply chains and drive valuable efficiencies and effectiveness, write Ralf Seifert and Richard Markoff
This article is republished with permission from I by IMD, the knowledge platform of IMD Business School. You may access the original article here.
In this era of digital supply chains and data-driven decisions, one could be forgiven for thinking that the most powerful and useful data a manufacturer of consumer goods could have when constructing forward demand forecasts would be current and historical consumer demand for their products. After a closer look with several planning experts, however, it seems that the real picture is more nuanced.
A complex landscape
Consumer product demand is often referred to by the term of art ‘sell-out’, to distinguish it from ‘sell in’, which is what a company might sell to a retailer, or ‘sell through’, which describes what a retailer ships to its stores. It is compiled from point of sale (POS) scans at retailers, who are, of course, the customers of consumer goods manufacturers.
But before considering how to best exploit it, one must sort out how to obtain it in the first place. The information must be purchased from third parties that collect it from retailers, for whom it is a source of revenue. The price will vary according to the number of references, locations, and retailers contained in the data, but can quickly run into hundreds of thousands of dollars.
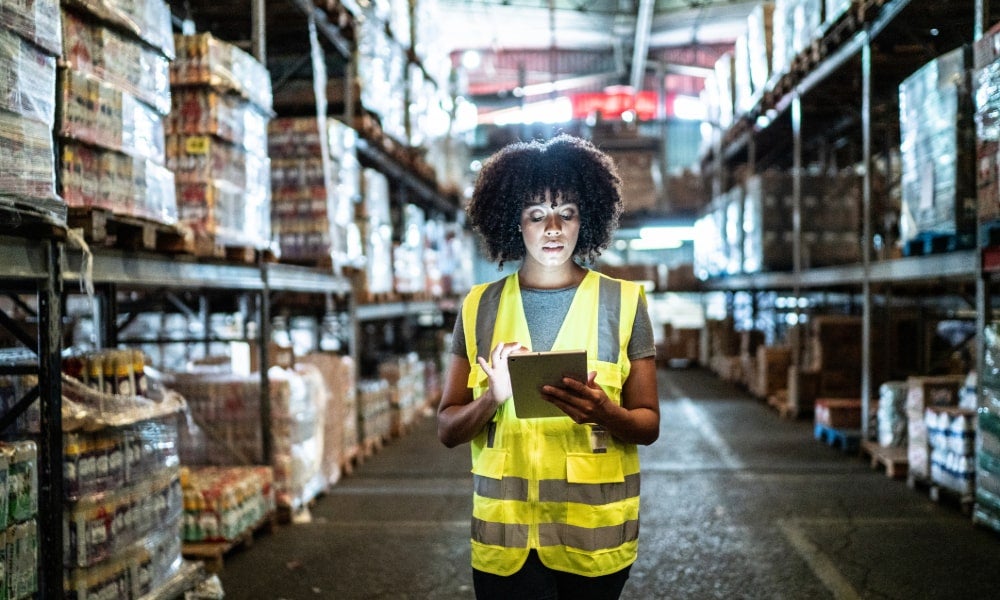
To make matters even more complex and get a complete picture, one would have to purchase sell-out data from several sources, since some have exclusivity rights with certain retailers, and some retailers have proprietary databases. And if that weren’t enough, some sell-out providers offer less granular packages but cover whole categories or offer insights into consumer profiles.
It’s worth pointing out that this minefield is avoided if the supply chain is vertically integrated, where the product is sold in ‘owned stores’ with complete access to the POS system.
After getting the data, then what?
There are real, non-negligible hurdles to properly exploiting sell-out data after purchasing. This starts with having the wherewithal to properly store, index, and manipulate the data. This is less of a problem for large organisations that have invested in data science resources. The next issue is that the data comes in very ‘messy’ and needs to be cleaned. Often, the EAN codes (the 13-digit codes embedded in the barcode) are mistaken or do not reflect product substitutions that occur with ever-increasing frequency.
A last wrinkle is that the data usually arrives in weekly tranches. Many manufacturers work in months rather than weeks, so a conversion has to be done. Just to add a final layer, there is no universal standard for which week is the first week of the year, so that has to be sorted out as well.
Read more: Why is excess inventory bad for business?
Putting the data to use
Only after the sell-out data has been purchased, compiled, cleaned, and converted can demand planners set about the task of using it to refine forward demand forecasts. Yet, it can be maddeningly difficult to accomplish this in any coherent way.
Demand forecasts at a manufacturer are the first step in the sales and operations cycle (S&OP). Properly done, the demand forecast will reflect the forward business activity and drive actions in the rest of the supply chain, including production. The implication is that the demand forecast must forcibly project the sell-in volumes that the manufacturer expects to sell to retailers. This, by definition, sets the financial plan and defines the demand against which the supply must be balanced and matched.
For a manufacturer to convert the information of what is selling at the retailer level into what will be sold to those retailers, one would have to reconstruct each step of the retailer supply chain. After all, a point of sale will increase its replenishment volumes from a retailer distribution centre (DC) if it is selling more, but for that DC to increase its orders to the manufacturer, thus impacting sell-in, a few things have to happen. First, the retailer must alter its store replenishment projections, and second, it must not be already overstocked. And for this to make sense, it would have to be modelled for each DC in a network for the stores that the sell-out data is sourced. To get a sense of the complexity, Walmart has 42 warehouses in the US, and it is just one retailer.
Only about half of manufacturers have advanced planning systems that fully model their internal supply chains, so it is not hard to imagine that this level of complexity is more or less insurmountable.
Read more: Three critical steps to an ethical and sustainable supply chain
Nonetheless useful for supply chains
This isn’t to say that sell-out data is not useful at all. One expert shared with us that they are experimenting with defining a ‘latency’ for core products. This is a rough-cut approach that seeks to define in broad strokes how many weeks a peak in sell-out will be manifested in sell-in demand.
Sell-out data can also be very useful for product launches, which ideally have been green-lit based on fundamental consumer demand. This gives a benchmark demand that can be compared to initial consumer sales and can provide a first glimpse into the success of the launch. This can enable anticipation of production without having to wait for the first replenishment orders from retailers. And since information silos still exist such that the bullwhip effect has not been eliminated, having insight into true consumer demand can help avoid misinterpreting sell-in increases.
Sell-out data has one more supply chain use case that is worth pointing out: it is one of the few reliable methods available to measure on-shelf availability (OSA). Using machine learning algorithms on sell-out data can help identify days when a product was likely not on the shelf and thus not selling as much as usual. This comes with limitations, however. It can be very useful for high-volume items like soft drinks or potato chips, but of little use for products that might only sell a few units each day, like hair dye kits.
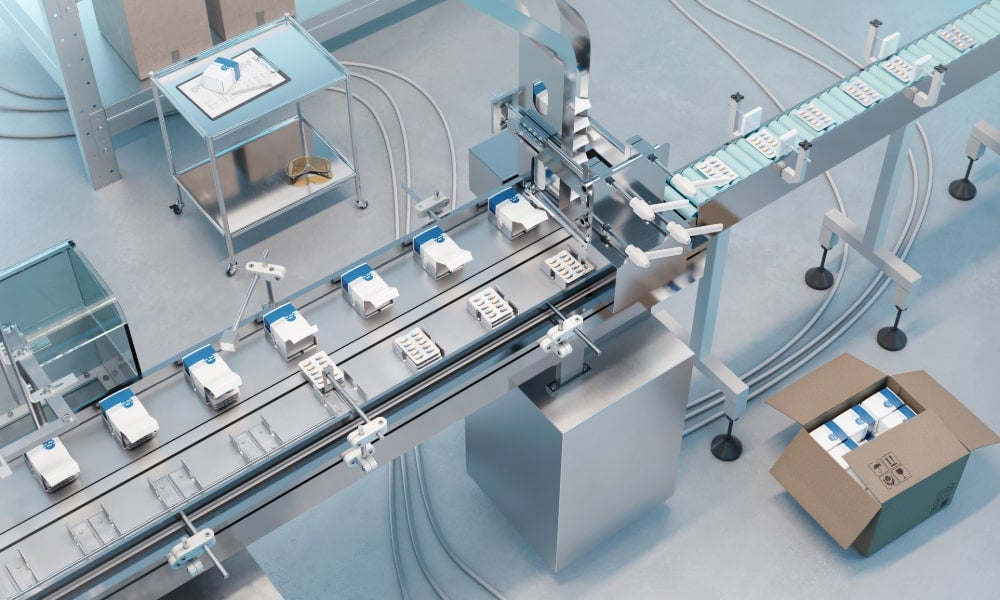
Outside the supply chain bubble
These use cases might not be enough to convince companies to justify the expense of purchasing the data and the investment in building capabilities to exploit it. The return on investment (ROI) might be a tough sell for supply chain managers to close.
When looking at a wider range of applications, however, sell-out data can offer critical insights into commercial performance at the B2B level. Sell-out data can help identify true sales uplift for promotional activity, helping to build P&L analysis for promotional efforts. Here, machine learning can also be a real benefit to demand planning, helping to size future promotions based on past sell-out performance.
Analytics based on sell-out data can also be a value proposition to retailers, helping demonstrate which stores are underperforming in launches or promotions. We have seen one manufacturer use sell-out data to reveal which stores were late in placing a new product on the shelves, an insight previously unnoticed by the retailer. There is a layer of irony to this since the manufacturer would be purchasing sell-out data, performing analyses, and offering this as a service to the customers where the data originated.
Subscribe to BusinessThink for the latest research, analysis and insights from UNSW Business School
Managerial takeaways
Although the first-order benefits of sell-out data-to-demand planning are difficult to harvest, there are second-order benefits worth considering. These additional use cases may be the difference in building an ROI to justify investing in sell-out data. It also has the additional advantage of being a vector for deeper collaboration between the supply chain and commercial teams.
And despite the great strides we are seeing in data science and artificial intelligence, challenges like using sell-out data, like so many others, are as much managerial and return-based as they are rooted in algorithms.
Ralf W Seifert is Professor of Operations Management at IMD and co-author of The Digital Supply Chain Challenge: Breaking Through. He directs IMD’s Leading the Future Supply Chain (LFSC) program, which addresses both traditional supply chain strategy and implementation issues as well as digitalisation trends and the impact of new technologies. Richard Markoff is a supply chain researcher, consultant, coach, and lecturer. He has worked in supply chain for L’Oréal for 22 years, in Canada, the US and France, spanning the entire value chain from manufacturing to customer collaboration. He is also Co-founder and Operating Partner of the venture capital firm Innovobot.