Five ways to figure out how to make the most of your data
Putting the right infrastructure in place and demystifying data analytics for employees will help companies gain a competitive edge, writes IMD's Amit Joshi
This article is republished with permission from I by IMD, the knowledge platform of IMD Business School. You may access the original article here.
Last year, organisations created 74 zettabytes of data, equivalent to a trillion gigabytes. To put this into context, an HD movie typically takes up a gigabyte of space on a computer’s hard drive. Yet only about 5 per cent of organisations end up making decisions that add value using this data
The reasons come down to a lack of workable data, as well as broader mistrust and fear of data among employees.
First, companies often lack the right infrastructure and tools to correctly organise the data they need. For example, they might want to send discount vouchers to customers to encourage them to buy more of a certain product, but the data on customer purchases and the data on pricing may be hidden away on separate servers. Without access to the right data, that is clean and well-structured, companies cannot go about analysing customer preferences or coming up with innovative new products.
Secondly, employees are often intimidated by data and wrongly believe that they need to be a computer programmer or data scientist to make sense of it. This is a myth. With the right tools and mindset, all employees can harness data to make decisions that add value.
As data analytic tools and machine learning technology become more prevalent and easier to use over the next decade, companies that don’t capitalise on the data they are sitting on risk being left behind and losing out. So how can companies make better use of their data?
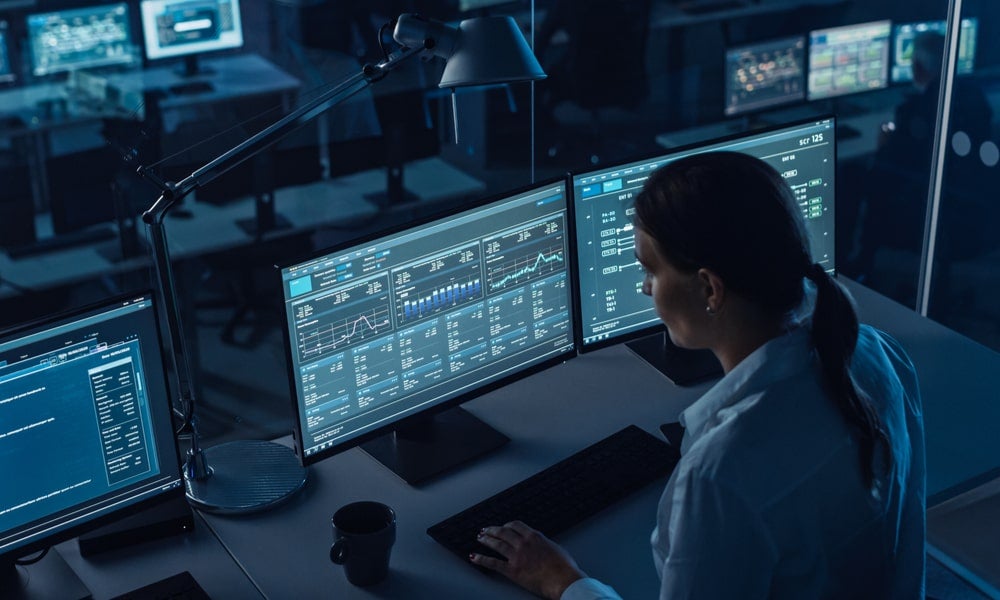
1. Invest in organising and cleaning data
Without appropriate processes in place to manage and sort data, there is no point in even getting started with analytics. The graph takes the “hierarchy of needs”, a model proposed by Abraham Maslow, the 20th century American psychologist, which offers a theory of psychological health predicated on fulfilling innate human needs in priority order, culminating in self-actualisation. Here we use this graph and apply it to the world of data.
At the bottom of the pyramid is collecting and storing and cleaning and organising data. Up to 80 per cent of a company’s time and resources are going to be spent down in the weeds, cleaning the data it has gathered, according to a survey of data scientists on Kaggle. This is unsexy but highly important work. As the saying coined by IBM programmer George Fuechsel in 1962 goes: garbage in, garbage out. If we put bad information into our computer models, we will get bad insights and make poor decisions.
Over the next decade, a proliferation of new intuitive tools and software will start to become available, enabling everyone in an organisation, regardless of their technical know-how, to work comfortably with data.
Read more: AI in the workplace: what should CEOs really be thinking about?
2. Identify the problem you want to solve
Too many organisations set about creating white elephant databases, which cost a lot of money to build, only for them to end up gathering dust on a server somewhere without any application.
As a first step, organisations need to think about the problem they want to solve and make the right data accessible to the people trying to solve that problem.
In my work helping organisations to use artificial intelligence and develop their big data, analytics and AI capabilities, I always advise clients to start off with several small quick-win projects. If you begin with a Big Bang data project, the chances of failure are high, and you risk demoralising staff. Instead, come up with a large portfolio of small projects where you should be able to see some results within six to eight weeks. If the problem you are trying to solve is going to take longer, try to break it down into smaller, more manageable parts.
3. Demystify data analytics
The second place where organisations stumble when it comes to deploying data efficiently is at the top of the pyramid, the so-called execution stage. Even companies that have the right data infrastructure in place may lack the ability to act on it. The good news is that most senior executives do not need to retrain as data scientists. Short courses and interactive training can help demystify the concept of data and give them enough knowledge to be dangerous. Business leaders should be able to understand the intuition behind data and identify where and how they can apply it.
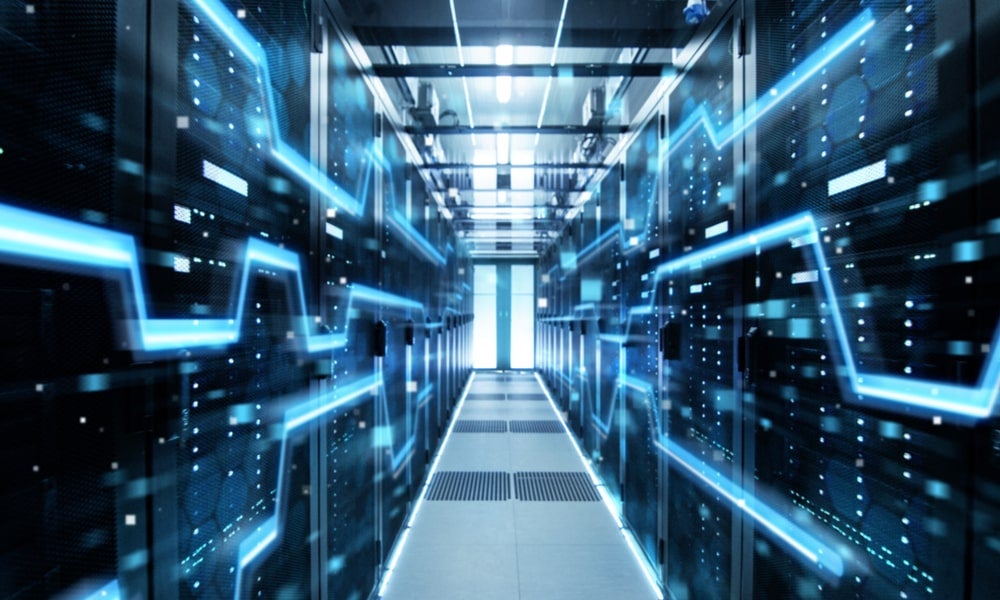
4. Hire people to interpret your data
To successfully deploy data to guide business decisions you don’t just need an army of data scientists (the numbers people); you also need to hire people who can translate the insights generated by your data into action.
A good data scientist will help you sniff out the right kind of data to solve your problem. But often the results might as well be written in Greek. A good business analyst will help you make sense of the numbers and turn them into practical insights such as, “We need to increase sales calls to four from three per month” or ”We need to change our pricing from x to y.”
Secondly, it is important that you create cross-functional teams that are using the data to solve the problem. Too often data scientists and IT departments will be sitting apart from the end-user. This is a recipe for building models that never get implemented.
One organisation that has successfully used big data and machine learning to improve customer experience at its theme parks and resorts is Disney. The company is estimated to have 1000 people, plus suppliers, dedicated to building advanced analytics capabilities and has deployed data to optimise everything from theme park logistics to ticket pricing and film production.
In contrast, an industry that has been slow to unlock the power of data analytics is banking. Firms continue to be surprisingly resistant to using data to drive decisions, preferring instead to focus on personal customer relationships with high net-worth clients.
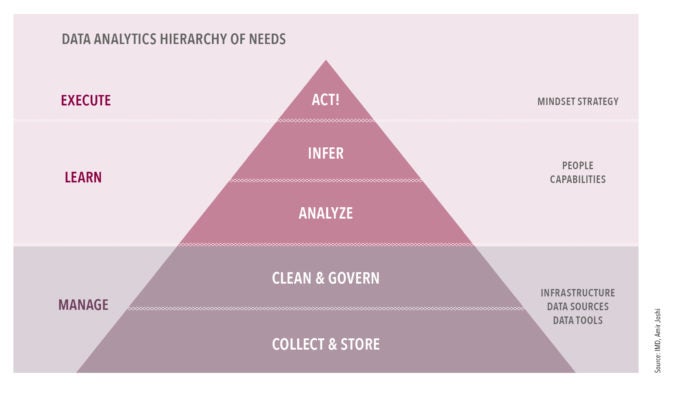
5. Pay attention to flawed or biased data
In recent years there have been several examples of companies developing tools and products based on flawed or biased data.
Amazon famously scrapped an AI recruiting tool that showed bias against women. The e-commerce giant had trained its computation models to vet applicants by observing patterns in resumes submitted to the company over a 10-year period. The problem is that most of those resumes came from men, who tend to dominate roles across the tech industry, meaning the algorithm learned to favour applications from men.
With customers and shareholders increasingly waking up to the risk of unintentional and potentially illegal discrimination due to the large-scale use of artificial intelligence (AI), the reputational risks for companies of failing to act on data in their possession are growing.
Property-sharing platform Airbnb came under fire for not doing enough to prevent discrimination after a 2015 Harvard study showed guests with distinctively Black names were less likely to be accepted than those with distinctively white names.
In addition, when using a third-party company or software for your data analysis, it is important to be careful that these firms are not relying on junk science. For example, there are several startups in the HR space that say they can use AI to tell if people have leadership skills by scanning a video interview when there is no scientific proof that this is the case.
Subscribe to BusinessThink for the latest research, analysis and insights from UNSW Business School
To avoid this trap, it is important to ask third-party vendors the following questions:
- How do you handle data biases?
- What are your ethical standards?
- How do you set up feedback loops so that you learn from what the market is giving you?
Over the next decade, a proliferation of new intuitive tools and software will start to become available, enabling everyone in an organisation, regardless of their technical know-how, to work comfortably with data. At the same time, a generation of digital natives who grew up surrounded by computers and digital devices and are comfortable interacting with AI-driven technology, such as chatbots, will swell the ranks of the workforce. These trends will force companies to unleash the power of their underutilised data if they want to remain ahead.
Amit Joshi is Professor of AI, Analytics and Marketing Strategy at IMD and program director of the Digital Analytics program, the Artificial Intelligence course, and the Business Analytics for Leaders course. This research builds on his 2020 paper (co-authored by Allègre L. Hadida, Joseph Lampel, W. David Walls), ‘Hollywood studio filmmaking in the age of Netflix: a tale of two institutional logics’, published in Journal of Cultural Economics (2020).