How Uber drivers revolt against algorithmic management
While algorithmic management offers operational efficiencies for companies like Uber, research has found it has also resulted in several real-world challenges
Ever wondered what it's like to be managed by an algorithm? Companies, especially those in the gig economy, increasingly turn to algorithms to oversee their remote employees, a practice known as algorithmic management. This approach has gained the most traction in gig economy work, with ride-hailing giant Uber as a prime example.
However, while algorithms as bosses can streamline management and decision-making processes, they can also introduce several unique problems. For example, Uber efficiently manages its workforce of around three million drivers through a mobile app that assigns passenger pickups and optimises routes. Still, it has grappled with issues such as worker classification disputes, allegations of bias and discrimination in decision-making, and concerns over transparency and worker autonomy.
Studies have demonstrated that algorithmic management can result in precarious work conditions, bias, and discrimination due to opaque, biased, big data-driven decisions. This situation can leave workers financially insecure and uncertain about their future. This can make them feel disempowered, as they often require human management for negotiation and guidance. And when workers feel disempowered, there’s a risk that they will revolt to regain some of that power.
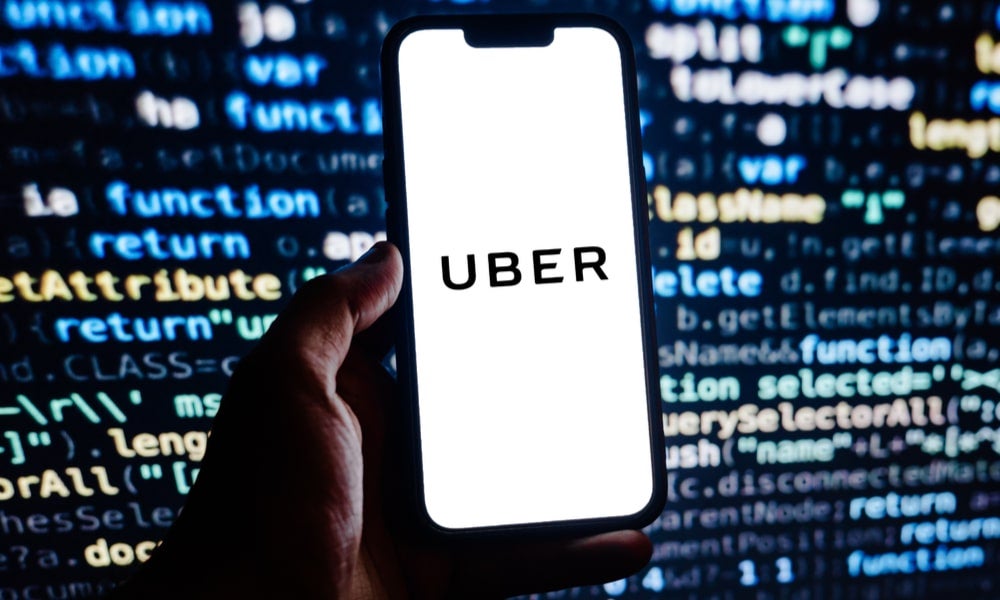
What are the problems with algorithmic management systems?
Because tech companies like Uber expanded rapidly, local governments have struggled to regulate and protect workers. So, to ensure a fair and equitable work environment where technology and humans coexist, the rapid growth of the gig economy demands a closer look at the issues related to algorithmic management.
This was the aim of a recent UNSW Business School study based on a PhD paper, Algorithmic management and the politics of demand: Control and resistance at Uber, co-authored by Emma McDaid, Assistant Professor of Accounting at UCD College of Business, and her supervisors, Paul Andon, Professor and Head of Accounting, Auditing and Taxation at UNSW Business School and Clinton Free, Professor and Deputy Dean at University of Sydney Business School.
McDaid, a former PhD student at UNSW Business School, set out to examine the construction and enactment of accounting control and information systems in the field of the platform organisation, using Uber as a case study. Her research won the 2020 Accounting and Finance Association of Australia and New Zealand Doctoral Award and the Dean’s Prize for Outstanding Research at UNSW Sydney.
So, what’s the difference between having a human manager versus being managed by digital platforms? A/Prof. McDaid explains that traditional management forms aim to motivate workers or address the complexities of their motivations. But when it comes to algorithms, the motivations are very different.
Read more: Steer it – don’t fear it: navigating AI with confidence
This means that gig work poses several unique workplace challenges, including reduced job security, decreased job participation, and – in the case of Uber – a focus on prioritising customers' needs over drivers. “Workers are not empowered in the same way as they would be in traditional modes of employment, but despite this, they act to resist this style of control by behaving in ways that contradict the algorithmic order,” she said.
“These actions range from subtle acts of defiance to more direct subversive acts of resistance, and they show the individual worker's need to reclaim autonomy and self-control in a job they are striving to endure. We conceptualise these behaviours as politics that arise from the on-demand economy called the politics of demand,” she explained.
So, while artificial intelligence and computing systems can streamline decision-making processes, they also lead to job insecurity. “Job security is sought by workers, as is having input in the job process(es). Having some level of discretion and autonomy in their work is often essential.
“New types of work emerge all the time, but with gig work, we see how a new value chain can be coordinated through algorithmic controls, but we also see how this impedes on many freedoms previously taken for granted,” explained A/Prof. McDaid.
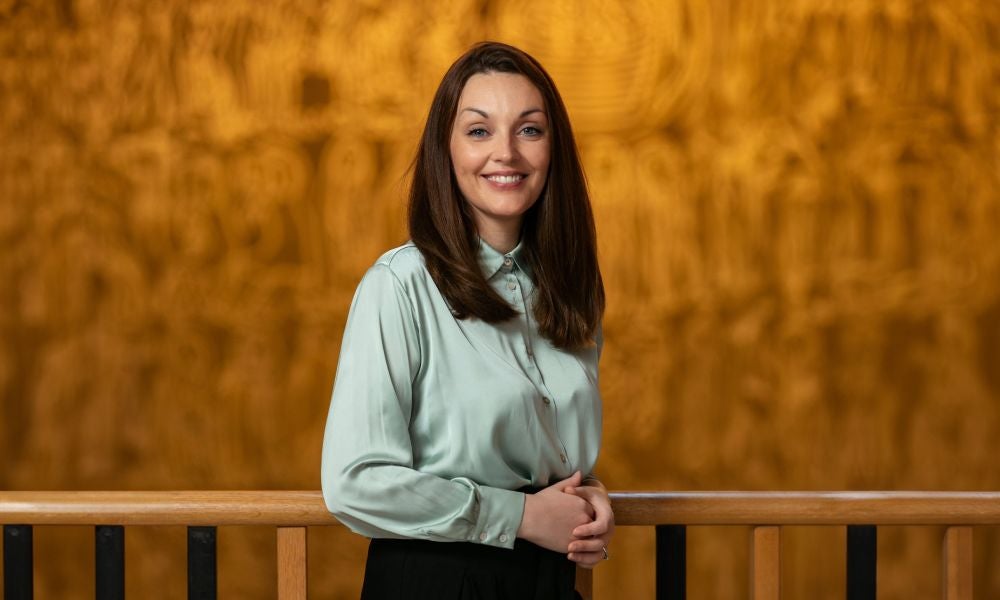
Why do Uber drivers rebel against algorithmic management?
According to A/Prof. McDaid, Uber employs five primary "algorithmic controls" via its driver app, including a 5-star rating system, GPS driving behaviour monitoring, rider allocation, dynamic pricing, and chatbot support.
Algorithmic control, or algorithmic management, uses computer programs to oversee and make decisions about work, commonly seen in the gig economy, where people work independently and remotely.
One specific algorithmic practice is passenger rating, where a minimum rating of around 4.5 to 4.6 stars is required, which disproportionately impacts new drivers as ratings are based on the last 500 trips. Deactivation becomes a real risk in the early stages if drivers receive several 4-star ratings.
But, according to the study's findings, these types of controls can backfire and lead some drivers to come together to work around them. For example, A/Prof McDaid described how drivers switched off and on the app at different times to confuse the algorithm or how often drivers would call customers for information the app would not provide.
Read more: Uber’s impact on traffic accidents is a lesson in calculating social benefit
“I learned through my PhD that many also used online forums to make new communities – I attended some early morning coffee catchups organised by drivers in the eastern suburbs. Many drivers use online forums, Facebook groups, Reddit communities, etc, to stay in touch for support. But these networks also help them strategies to work most efficiently within the algorithmic control environment,” she explained.
But these trends don’t just impact gig economy workers. A/Prof. McDaid explained how many employers can now use technology to gain more insights into employee behaviour than ever before. “For example, employers can know what employees do in real-time, which software they use, the keystrokes they deliver per minute, etc. Technology is changing work and workplaces, and its power in the Uber case study exemplifies that," she said.
“But while organisations have used surveillance over workers for years, we now need to balance that need for management supervision with the risk that we could dehumanise workers in the process.
“The evaluation of someone's work requires context, and as technology cannot provide that context, there is the risk that people will be unfairly evaluated and managed inside and outside of the gig economy. It is a general worrying development and requires attention in workplaces of all types."
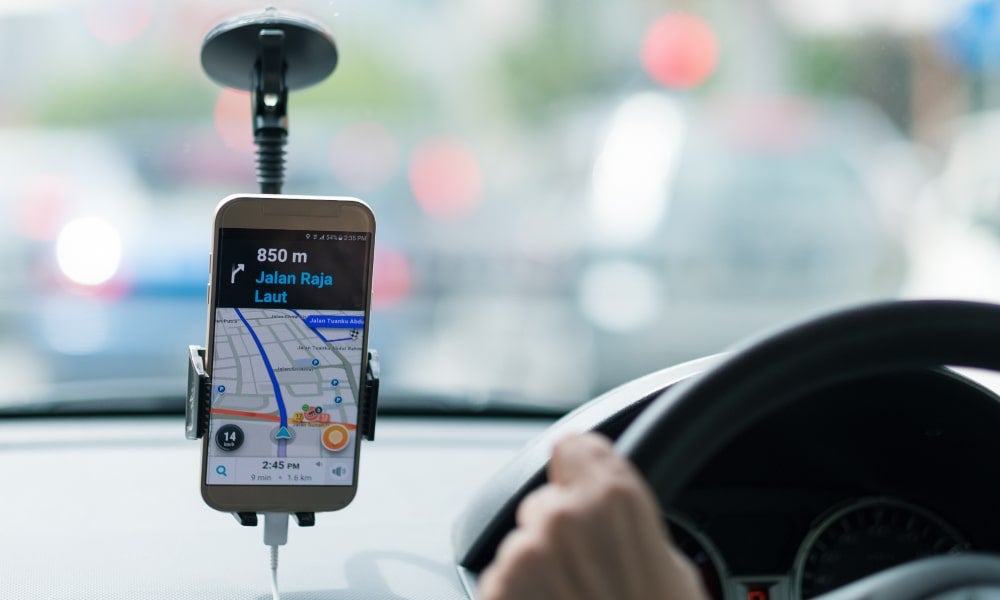
How to improve algorithmic management
One area where algorithmic management can benefit from evidence-based insights is performance metrics. For example, A/Prof. McDaid explained that the driving style dashboard provides drivers with green ticks, amber dots or red x's depending on how drivers are 'driving' out on the roads. Many participants needed clarification on the timeliness of this control and the consequences, for example, of repeated red x's.
“Based on my research, platforms should involve workers in the control design or measure setting process. This would enable the platform to understand what is controllable and make the measure more effective,” she said.
“As part of the resisting behaviours noted in the paper, many drivers were starting to build private transport businesses through the customers they met via gig work. Many also installed dash cams in case they wanted to add ‘context’ to the version of events reported by the algorithmic systems that monitored their physical movements,” she explained.
So, what can help improve outcomes for organisations as well as employees? There is a need for more transparency around the design of algorithmic controls and, linked with that, better communication established with workers around algorithmic rules.
Subscribe to BusinessThink for the latest research, analysis and insights from UNSW Business School
“Involving workers in the measure setting process would significantly improve their experience of gig-work. Allowing for cross-cultural behavioural differences is essential, as habits and customer behaviour can vary depending on the region.
“Algorithmic controls should be adaptable too – could organisations like Uber flex their controls for drivers who have been with the platform for a long time, providing a more tailored control environment based on the driver profile? This would resolve the issue of the controls being 'too tight' for drivers who are beginning to work with the platform,” she concluded.